In this section
TIMBUS Partners
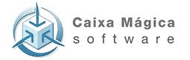
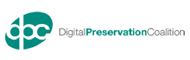
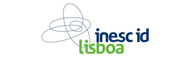
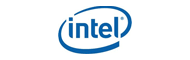
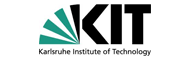
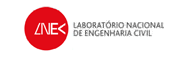
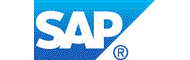
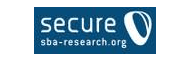
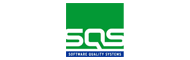
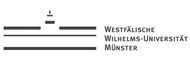
Preservation and Re-Deployment of Sensor Data in a Civil Engineering Use Case
- Details
- Created on Thursday, 07 November 2013 16:54
- Last Updated on Tuesday, 21 October 2014 08:32
|
Anja Bachmann, KIT; José Barateiro, LNEC "The most promising method to resilient re-deployment we propose in this work is based on a model that was produced by using machine learning algorithms that were trained with large amounts of historic sensor data. It can not only be used to reproduce past and predict future behaviour, but also to detect anomalies." |
|
In the first edition of the TIMBUS newsletter, we discussed why large, long-lived infrastructures, such as dams, especially need resilient processes surrounding them. Associated processes have to be monitored to ensure that they remain continuously accessible in a changing technology context. In our civil engineering use case we consider the problem of digitally preserving dam safety monitoring processes supported by a safety information system. Digital preservation is a crucial element in ensuring structural safety by
- Evaluating and validating measurements and their correlations
- Interpreting past and predicting future behaviour
- Estimating missing values and detecting anomalies
This enables contextual interpretation even decades later.
Figure 1: A group of five extensometers before their installation, which is done during the construction. (Source:LNEC)
There are three kinds of data that are relevant as well as different storage methods.
First, we have sensor data. This consists of the sensor readings, i.e. all measurements acquired by the sensor, and meta-information about the environment that influence these measurements, e.g. average temperature or the water level within the dam, both of them influencing the extensions measured by an extensometer sensor. Electrical extensometers are embedded inside the structure and thus, cannot be replaced. Figure 1 illustrates such an extensometer. It is one of many possible sensor types and the one we are focussing on in our use case. So far, this information is stored in an Oracle database that can easily be accessed by different tools.
Second, we have meta-information about the processes the sensors are involved in. Among others, this consists of dependencies between the processes, their inputs and outcomes and their risks. Such information is stored in a general TIMBUS ontology that captures all business process context information.
Third, there is also information about the dependencies and properties of a sensor. This consists of general sensor information, such as sensor type or structural location, but also acquisition information, such as acquisition rate and quantity of the measurements. Additionally, sensor calibration parameters and conversion parameters are stored. The latter are used to convert the raw readings into physical quantities; in our use case, the extensometers total resistance and a relative resistance (relative to a former reference point) are converted into temperature and the actual extension of the sensor. All this is stored in an ontology that was created especially to describe sensors, their meta-data and properties.
Ontologies are a suitable storage format for contextual and meta-data as they enable defining properties and dependencies between concepts due to their triple-store property. A visualization of such an ontology also supports interpretation of processes and dependencies.
Figure 2: Dam safety processes and their information life cycle: using TIMBUS to improve the preservation capability. (Images:LNEC)
The relevant data is extracted and then preserved within the mentioned storage type. The measurements are acquired directly from the sensors (either automatically or manually by a reading device) and then transferred to the database. This database also contains meta-information about the dams, the sensor location and the quantity of the measurements. The latter is important to build the sensor ontology. General information about the processes and the risks was gathered by analysing the system (in part supported by experts) and by extracting information using TIMBUS context extraction tools. Figure 2 illustrates this.
The preserved system can be re-deployed and measurements can be replayed in the context of the preserved processes. TIMBUS project partners LNEC and KIT evaluated several methods to serve as a blueprint for future realizations of holistic preservation strategies. We published a first analysis on the 5th International Conference on Knowledge Management and Information Sharing (KMIS 2013). The most promising method to resilient re-deployment we propose in this work is based on a model that was produced by using machine learning algorithms that were trained with large amounts of historic sensor data. It can not only be used to reproduce past and predict future behaviour, but also to detect anomalies.
Novel approaches to digital preservation are important in the context of monitoring large infrastructures like dam systems. They enable contextual interpretation and retracing of the past. Such methodology, as developed in the context of the TIMBUS project, brings civil engineering a step closer to risk mitigation and failure avoidance. Future work (on which we will report here soon) contains the development of an extractor to support transformation of data into a canonical ontology-specific format. Additionally, we will implement and evaluate the redeployment algorithm and a corresponding framework. We will evaluate different redeployment and anomaly detection techniques for various sensor types with respect to their applicability in the context of digital preservation.