In this section
TIMBUS Partners
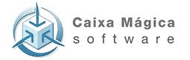
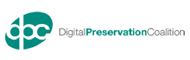
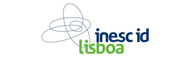
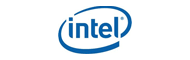
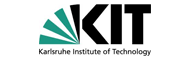
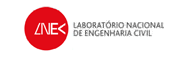
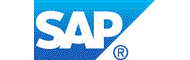
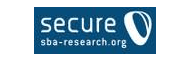
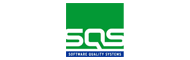
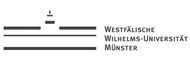
Time-Series Annotation and Context Extraction Tool
- Details
- Created on Tuesday, 27 May 2014 09:25
- Last Updated on Tuesday, 21 October 2014 08:32
Anja Bachman (KIT) "We are happy to announce the open source release of the Time-series Annotation and Context Extraction Tool (TACET)" |
|
In the context of a Civil Engineering use case and as part of the TIMBUS Extractors, we are happy to announce the open source release of the Time-series Annotation and Context Extraction Tool (TACET). Its main purpose is to import time series monitoring and sensor data and annotate it with different labels, e.g. activities, status information or expert knowledge. This data can then be used as input for classifiers to predict future values or detect anomalies.
The tool is available for download from opensourceprojects.eu.
Figure 1: Screenshot of the TACET main window after importing extensometer data. At the top it shows the "Annotation" tracks, here indicating normal or abnormal behaviour and marking the time series interval to be used as training data in the classification. Below, the "Sensor" tracks are shown, in this case representing the quantities "resistance", "temperature" and "extension" of an extensometer sensor and the ID of the reading.
Each dataset will be displayed as a sensor, logging, annotation, context or video track and be processed in parallel. Different measurements can be labelled in combination. Examples of this include sensor values gathered by an extensometer. This facilitates the interpretation and extraction of context parameters. External data streams and video an be used to support such an observation.
The resulting data can be used as input for different data mining plugins (based on WEKA). Data Mining tools offer the possbility to classify or cluster data to context information. Classification is a technique that is often used in Machine Learning to train a model. Concept Learning is one example of this. The classifier processes input data with all its features and attributes and learns the corresponding class attribute (label). Later on, the model is fed with new, unlabelled input data. Based on the training data, the new data is then classified. We are currently working on an importer for data from the TIMBUS context model or DSO and corresponding exporter to populate the annotations.
Another application is for anomaly detection. One option is to feed the model with historical sensor and context data during the training. If incoming data is later classified as abnormal, the user has to check whether it is sensor damage or indeed and anomaly, such as a water dam failure. Another option is to define constraint and threshold rules. Detected anomalies either reflect faults and failures or deliberate process changes that indicate a new version of a process. Within a defined process, preservation actions can be taken.
The tool supports various input and output sources, and is extensible to a wide range of use cases: possible cases include the usage of the tool with sensor data from other large infrastructures (such as atomic or electrical power plants or bridges) or in wireless sensor networks. Using an open source development model, it can be further adapted to specific use case needs.